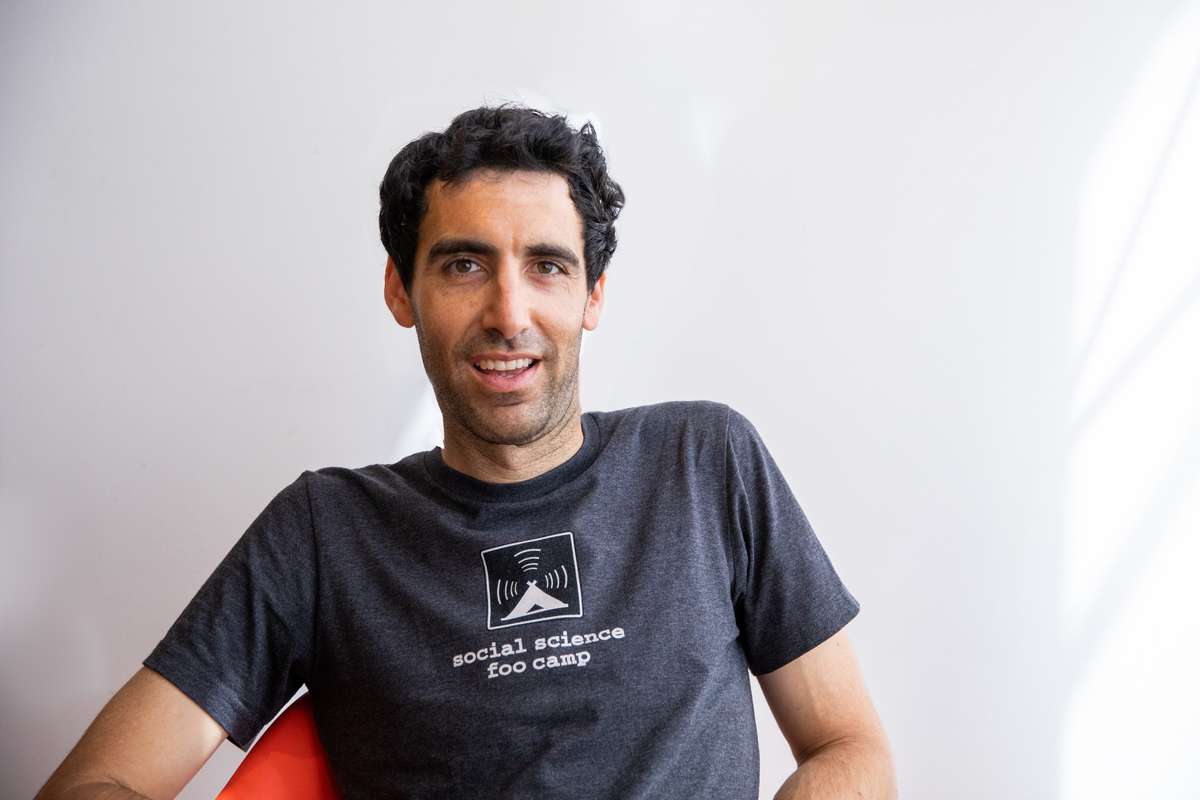
What are the core components of a strong data scientist skill set? And how should you evaluate a data scientist candidate once you find them? HackerRank CEO and Co-founder, Vivek Ravisankar, sat down with Kaggle CEO and Co-founder, Anthony Goldbloom to explore the ins and outs of this growing role.
What do Lyft, the Radiological Society of North America, and Booz Allen Hamilton have in common? All three rely on Kaggle to answer some of their biggest data science and machine conundrums.
With over 3.8MM users, Kaggle is the world’s largest data science and machine learning community. It’s home to 25,000+ public datasets, nearly 300,000 public notebooks, and a library of data science micro-courses. Through their data science competitions, they encourage their community to tackle real-world machine learning problems across industries.
As CEO and Co-founder of Kaggle, and as a data scientist himself, Anthony Goldbloom is one of the foremost experts on the exploding field of data science.
Though it’s now one of the fastest growing fields in the world, data science is still young. In fact, the term “data scientist” as we know it wasn’t coined until 2008. But the growing application of data science across industries has led to an uptick in demand for data scientist talent. And that's made it a hot topic amongst employers.
Its rapid rise to popularity has led to confusion amongst hiring teams. What skills does a data scientist have? What do they work on? And what’s the difference between data scientists and machine learning engineers?
To understand through the eyes of an expert, we sat down with Anthony to learn how data science as we know it came to be—and what skill set defines a “data scientist” today. Here’s what we learned:
2012 and 2018 were defining years for the field
According to Anthony, 2012 was an annus mirabilis (or, a “miracle year”) for deep learning. With the introduction of neural networks, machine learning and artificial intelligence (AI) took off. That lead to a landslide of advances in natural language processing, speech, and computer vision. It’s why we’ve seen such a boom in applications of computer vision across cases like self-driving vehicles, radiology and security cameras.
Thanks in part to those advances, neural networks and gradient-boosting now play a major part in defining the day-to-day of a data scientist. “Those are the two things I think data scientists today are spending most of their time on,” Anthony said.
Hear the extended audio interview on our podcast, HackerRank Radio:
[powerpress]
But advances in 2018 may change that focus moving forward, according to Anthony. “You could argue that 2018 was the annus mirabilis for natural language processing.” With new advances in natural language process, we’re bound to see an increase in use cases.
“Just as autonomous cars, and radiology, and some security use cases have been unlocked by computer vision—what sort of use cases might we see around natural language processing?” Though only time will tell, teams may see those use cases impact hiring needs moving forward.
The role "data scientist" has many meanings
Within data science, there are a number of distinct roles, from data analysts, to data engineers, data scientists, and more. But the difference between them isn’t always clear amongst employers. “I’m not surprised that companies are confused—because it is fairly confusing.”
When it comes to distinguishing data scientists from data analysts, Anthony’s criteria is simple. “I classify a data scientist as someone who writes code in order to produce inputs.” A data analyst or business analyst, on the other hand, leans primarily on tools like Tableau or Excel. A data scientist’s role can also span a wider set of responsibilities. “[A data scientist] could be doing anything from writing pivot tables all the way through to training machine learning models.”
But even the term “data scientist” has its own set of nuances. To Anthony, “data scientist” is an umbrella term that can be used to describe a variety skill sets and focal areas. In his eyes, there are 2 primary subcategories: type I data scientists, and type II data scientists.
Type I data scientists
Type I data scientists focus on building algorithms that will go into production. The algorithms that power Facebook's newsfeed and Netflix's content recommendations are good examples.
As a part of their work, most type I data scientists spend their days training a machine learning algorithm (often, either a neural network or a gradient-boosting machine). This is the category where Anthony places Machine Learning Engineers and AI Engineers.
Type II data scientists
The work of type II data scientists, on the other hand, generally isn’t destined for production. Instead, this type of data science focuses on deriving and analyzing insights that a business can ultimately productionize. “It’s insights that you can productionize,” Anthony says.
A type II data science may use similar tools to a type data scientist, like machine learning algorithms. But they may also be working on something as simple as a pivot table.
A strong data scientist skill set isn't just about technical know-how
No matter the subtype of data scientist, one thing is clear: technical acumen alone doesn’t an effective data scientist. Instead, a well-rounded data scientist has a combination of both hard and soft skills, including (but not limited to) technical skills, business acumen, creativity, and communication. So hiring teams need to look for much more than technical expertise.
Underlining the importance of business savvy
Anthony has seen this firsthand through competitions on Kaggle. “People will build an amazing algorithm on [a] problem,” Anthony explained. “But if the evaluation metric is wrong, or the target variable isn’t a variable that’s useful to predict, then the algorithm is completely useless.”
Understanding the algorithm’s ultimate application is the difference between a functional, but ineffective algorithm, and one that solves a real problem. Even the most technically sound algorithm—if designed to produce a meaningless output—won’t yield a desirable result. “Building an effective algorithm requires you to be strong technically, but it also requires you to have good business context.”
To produce good work, a great data scientist needs to know how their work adds value to the company, and ultimately, how it will be used.
How Anthony's team evaluates Kaggle profiles (hint: it's not about competition scores)
So, how does Anthony’s team find data scientists with this unique skill set? For starters, they turn to Kaggle. “Our community’s actually a very good signal.”
But reading a Kaggle profile isn’t as intuitive as you might think. “We grade people on 3 criteria: their competition performance, the kernels [and notebooks] they share—how many upvotes they get—and their contribution to discussion [in the forums].” Typically, a candidate that’s done well in any 1 of those 3 areas will get an interview.
That said, it doesn’t mean that all criteria are created equal. “Of the 3 criteria...I care about most about discussion,” Anthony said. Why? Because it’s an indicator of both technical and soft skills. “You only get upvotes if you are technically insightful and you’re a clear communicator.” But in competitions, for example, you can be technically strong with poor communication skills—and a strong data scientist skill set requires both.
Where the most successful data scientists come from
Data science is still a burgeoning field—so candidates that come to data science from diverse backgrounds are something of a norm. With the right mix of technical expertise, curiosity, storytelling, and cleverness, people from virtually any field can become a data scientist.
In fact, an analysis on graduates of the Insight Data Science Fellows Program—a training fellowship designed to help PhD graduates transition into data science—showed that successful fellows stemmed from fields ranging from Physics to Neuroscience to the Social Sciences.
Of roughly 700 Insight Data Science fellows analyzed, graduates came from a variety of academic backgrounds ranging from Physics to Social Sciences (via Scott Crole)
Given the variety of backgrounds they come from, resumes and verbal interviews aren’t always the most effective way to evaluate the skills data scientists have to offer. When it comes to hiring, Anthony has had the most success evaluating data scientists through miniature projects.
“[We’d] give them a project that we cared about, or that would look like a project they’d tackle internally,” he said. For Anthony, it gives a more nuanced look into their skill set as a data scientist. “We learned more from that than anything else, frankly.”
The project-based approach didn’t just spotlight their technical skills. By asking candidates why they made the decisions they did, it also gave the panel an opportunity to explore soft skills like business savvy, storytelling, and communication. “And I think there’s probably just about no substitute for that—because a good data scientist can come from a very wide variety of backgrounds.”
Evaluating data scientist skill sets
Data science is an evolving field—and like any new field, it’s going to align on the nomenclature, the use cases, and the skill sets that define it. But by diving into the history of the field—and the way it’s applied today—you can better understand how to hire them.
Hiring data scientists at your organization? Read more about HackerRank Projects for Data Science, or read what we learned about data science hiring from our survey of 70,000+ developers and technical professionals: